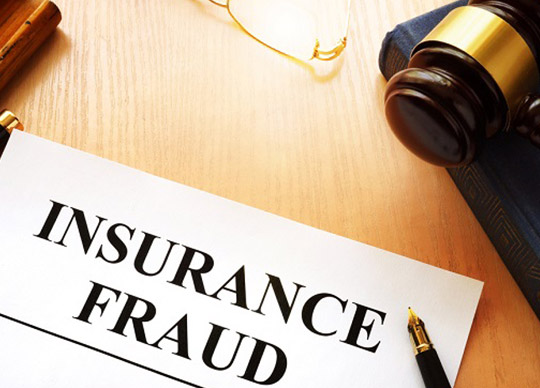
Fraudulent claims are a key driver for increased insurance premiums, prompting a data analytics company to suggest ways to reduce the burgeoning costs.
The Insurance Fraud Bureau of Australia, part of the Insurance Council of Australia, estimates fraud costs the insurance industry up to $2.2 billion annually.
Data and analytics specialist PBT Group says the foundation of reducing fraudulent claims is to understand fraud trends using claims and underwriting data.
Nitin Mistry, Melbourne-based PBT Group’s Senior Data Analyst, says organisations with a higher level of data maturity can identify and prevent fraud more efficiently.
“While artificial intelligence (AI) and machine learning (ML) can automate fraud detection, several factors impact on the accuracy of AI/ML models, such as data quality and variety. However, organisations can overcome those challenges by improving processes and incorporating external data,” he says.
“Building and implementing an AI/ML fraud model is not an overnight, one-off exercise, it is an iterative process in which collaboration between technical and business teams within the organisation is critical to achieving success.”
Mr Mistry says an analysis of Medicare uncovered a host of data issues that are likely replicated in many insurers.
The Federal Government commissioned health economist Dr Pradeep Philip to review the integrity of Medicare and its health provider compliance mechanisms.
Dr Philip’s March 2023 report found a lack of capability to complete sophisticated analytics to identify areas of risk, including fraud. Medicare had insufficient “capability maturity in systems and processes to enable … a proactive, prevention and early detection-focused approach”.
Implementing an analytical model would capture most adverse behaviours early and deter opportunistic and organised fraud, Dr Philip said.
Mr Mistry recommends insurers initially conduct a data heath assessment to determine whether their data is sufficiently mature to enable an AI/ML fraud detection model. 2
They then need to identify the business need for a model; determine the goals, benefits and risks; design an implementation plan; then monitor the model’s performance and financial benefits.
“Fraudsters don’t have just one page in their book, so it’s essential to keep up to date with their scams and retrain the model accordingly,” Mr Mistry says.
Insurance fraud includes exaggerating legitimate claims, intentionally misrepresenting the facts, or complex, organised manipulation of the claims process to gain financial advantage.
AI and ML use algorithms that can identify patterns specific to fraudulent activity. The tools help humans review large amounts of data more efficiently, enabling them to detect potentially fraudulent claims early.
However, Mr Mistry says while AI and ML minimise human interaction in fraud detection, human oversight is essential to ensure the model’s accuracy and reliability.